Curing the Property Pain Chain
InsTech Podcast with Hemant Shah:
Curing the Property Pain Chain
We’re thrilled to have had our co-founder and CEO, Hemant Shah, join Matthew Grant on Episode 207 of InsTech London’s weekly podcast.
InsTech London identifies and promotes the use of the best technology, data, and analytics within insurance and risk management around the world. The company provides insights through events, podcasts, reports, and a powerful network of industry professionals.
The podcast's talking points cover:
- How Archipelago is working with companies to collect information about properties for insurers and brokers
- Issues associated with matching loss history data to exposure data
- How exposure data is described, validated, and standardized
- The potential role of intelligence augmentation and the process behind implementing machine learning
- How ESG requirements are changing resilience reporting
Read the InsTech London Podcast Episode 207 transcript or listen along below.
Curing the Property Pain Chain
Episode 207 transcript:
Matthew: Good morning, good evening, wherever you are and whatever you're doing. Matthew Grant here and welcome back if you are a regular listener. If this is your first time, well, I'm delighted you have found us. Well, we're going to be talking about property in a minute but first of all, news from Norway in the last couple of weeks that 9 out of 10 people having accidents at nighttime on e-scooters were drunk. Well, another one for our list of uninsured and uninsurable, I think.
But back to today's guest, Hemant Shah, who, having successfully tackled the problem of modeling earthquake and hurricanes, launched Archipelago to help insurers and their clients figure out what the world's commercial properties are actually made of, where they are and who they belong to. Not as easy as many people might think. Well, we are delighted that Archipelago is one of over 170 companies we are working with now at InsTech from both insurance and technology large and small.
Like many of the companies we know and many of our guests, Archipelago has a significant presence in the US as well as the UK and around the world, so if you're working for Insurer and want to know more about the companies with the solutions to your problems or if you are one of the companies solving those technology and analytics problems, then please let us know, hello@intech.co or drop me a note, Matthew Grant on LinkedIn.
Now, finally we know many of you are listening quite a lot, by the way, but we don't know who you are or what you think unless you tell us. We enjoy talking to the many of the fascinating leaders and founders around the world, but it makes it even better when you tell us what you like or what you'd like to see more of. Matthew Grant on LinkedIn should do it. Now, over to Hemant for today's slightly extended version.
Hemant, I have been really looking forward to having our second discussion. We'll talk a bit more about our previous one in a moment, but for those that haven't come across Archipelago yet, just a few words about what you're doing. I'm sure you'll add a bit more to this. You founded it back in 2018. You're working with large global corporations to identify and collect key information about their properties. That's then shared with insurers and brokers. I believe you've got over 170 people, and according to our friends at PitchBook you've raised $57 million. You're co-founder and CEO and, of course, you're also co-founder and CEO of catastrophe modeling company, RMS, where we worked together for 20 years. So good morning, nice to be talking again.
Hemant: Good morning, Matthew. It's great to reconnect with you. I'm looking forward to this conversation.
Matthew: Well, you've got to catch up or exceed, I think, actually, your number of downloads from last time. You made it to our 1000 Fans Award. You've actually... I've been looking at data. You've got 1303 downloads. You actually had 20 in the last month, which just shows that it's sort of a podcast that keeps on giving. You also had Anthony Siggers on episode 162 when he was talking live on stage. Yeah, anybody that wants to catch up on you again after this, 145, but it's great to have you back. We're going to be talking about what's changed in the last year.
Hemant: When we last chatted, Matthew, we were exclusively focused on platforming SOV data, exposure data about the property, the detailed attributes, the COPE information, the values, the primary and secondary modifiers, all evidence linked from primary source documents. What we've now expanded the platform is to the second key data type, which is not just the independent variables of the property, the roof systems, sprinkler systems, values, construction, but what has happened to those buildings in terms of incidents and claims. We now are onboarding loss history data, matching it uniquely and immutably to the property exposure information, which is powering an additional set of insight about the exposure and risk through the lens of not only the exposure data, but the claims data associated with those properties.
Matthew: That's really powerful. I want to come back and talk about that. Before we do, just because you threw out two acronyms there, SOV (statement of values) and COPE. I'm going to get halfway through that. I'm going to forget what they are. Construction, occupancy... You've got to help me with these other two.
Hemant: Protection and exposure
Matthew: There we go. Team effort. We got there in the end. Yeah, I mean one of the problems, of course-
Hemant: You can share that at the next cocktail party you're at, Matthew. It will be a big hit.
Matthew: Well, if we start talking about climate we're going to get into five letter acronyms so watch out. You just need to be on board for those. But, of course, one the problems you're kind of solving there or helping people with is often underwriting information, claims information live in two completely different worlds and they actually... It's quite difficult to reconcile claims information. It sounds like your clients are now willing to share those with insurers and brokers. I'm sure there's some constraints on how they do that, but how's that going down with the insurers?
Hemant: Well, the data is shared. Loss runs is the term of art for the claims information, and that's a core part of every submission – which is the historic loss experience on the insurance portfolio, often specifically at each property level. Now, the challenge is, like the statement of values, the exposure data, that also lives in a spreadsheet and so typically while underwriters will receive an email from their broker with a request to quote, there'll be a number of spreadsheets and PDFs that describe the exposure data. But then there's also spreadsheet or spreadsheets that describe the loss experience on the portfolio.
What we do on Archipelago is connect this data uniquely at the property level so you can analyze and trend not only what the aggregate loss experience has been, but how the current portfolio and its history relates to the historical claims and compare the two to each other, including the reconciliation on what historic claims are still associated with properties that are still in the portfolio. Often these portfolios change and the historic claims experience may be less or more relevant to the current portfolio than it was to the past. Those are difficult. It's not rocket science because the information exists, it's just all disconnected and living in different spreadsheets coded differently and it makes it hard to compare trend and analyze. We make that easier. Our customers are already sharing their loss experiences with their brokers and their markets in order to get the quotes. Now they can integrate how they share, on Archipelago, the exposure data and the claims data all in one place, all connected, all trended and all analyzable – not only by them, but by their underwriters as well.
Matthew: And knowing you, I'm sure you're not extending the life of the technology that just shares spreadsheets between different parties. What technology have you got that is... That information consistent or is replacing spreadsheets?
Hemant:
It's about the data and it's about standardizing the data. It's about enriching the data. It's about evidence linking the data. It's about making multiple years of the data all accessible and comparable. And it's that underlying technology is immutable so we don't override information. Everything is maintained and connected so you can see the life cycle and time histories of all these properties.
It's based on a very powerful sharing and permissioning architecture that allows this data not only to be consumed by the risk managers and their organizations internally, but you can invite in their broker partners who can then invite in the underwriters to essentially virtual data rooms to consume the information. It's more than just the spreadsheets, it's about how the information is represented, how it's validated, how it can be analyzed, and how it can be shared across the ecosystem of all the various counterparties who need access to this information to collaborate and to underwrite and price the risks.
Matthew: The, not very exciting, but the bit always engages people is what about the... You touched on in there, but the kind of consistency of the format and the data's exchange. One of the big challenges here seems to be figuring out who is ultimately going to drive that consistency, but I assume as part of what you're doing is actually creating a more consistent standard for how the data is being exchanged and absorbed.
Hemant: Yeah. The current process, which we often refer to as the pain chain, has a lot of pain points associated with it, but it is remarkable that there are some real basic pain points that really are persistent and persist throughout this whole process. You flagged one of them. Irrespective of how complete the information or irrespective of how accurate the information is or how well connected the time histories of the information are on unique IDs over time, there's a consistency problem.
Every single spreadsheet that an underwriter receives is formatted in a different way. Every spreadsheet, even if it's a consistent format, often has different coding standards.
Sometimes the information's recorded in one model format versus another model format, sometimes it's a free text description as in, "This building has a glass curtain wall." They'll write a sentence into the field.
These inconsistencies are not only making it difficult for the underwriters and their teams to discern what is the actual characteristics of the property, it's very difficult for them and time-consuming and often they can get it wrong.
It's like a game of telephone where at each hop in transformation, somebody might make a different interpretation of the construction of the building, the roof system of the building, the fire protection systems of the building, and these inconsistencies are grit in the entire system that blocks cognitive analytical processing on the data in a consistent fashion.
Yeah, so the consistency of the inconsistencies are a key pain point. One of the many benefits that we deliver is a consistent, standardized coding that can support transformations to multiple insurer models and analytic tools.
Matthew: Yeah. It's a topic we all hoped would've been solved by now. It sounds like you're doing your bit to improve it. Hopefully we'll no longer be having that conversation in a few years' time, although... I don't know if you saw this, we did a survey of 170 companies we're working with of what they felt the main topics of interest were both for insurers and technology companies. Top three on the list was the ability to extract data from PDFs. I mean, it's still... We're not quite where Insurtech thought it would be seven years ago trying to figure out how to get data from PDFs.
Hemant: You mentioned PDFs. One of the core insights that we've gained by working with these large owners, these corporations who buy considerable amounts of commercial property insurance, is that there are very compelling sources of truth that describe the facts of the property, but often they are locked up in documents – PDFs, Word documents, schematics, photographs.
A lot of our tech can extract data out of these unstructured data sources. You can define a set of machine learning to process those documents to extract the pertinent information and then link the document to the data to create a sense of providence and evidence like a lineage to where the data came from, which builds confidence that the data is actually accurate. It's kind of like having a virtual inspection of the property.
Matthew: How often does someone need to be in the loop on that because, I mean, one of the themes that I've seen coming quite strongly in the last 12 months is a recognition that AI, artificial intelligence, can go so far but there's this new term coming in called intelligent augmentation, which is where the... I mean, I'd often describe it as the AI needs to know when it doesn't understand or flag it. It seems like there's going to be a role of humans in that loop for a long time but I don't know you can put a figure on what the percentage is of when somebody has to look at it versus automating it, but what's... I mean, and also direction of travel, I guess, because as your own analytics get smarter and hopefully the data gets slightly better at source, what should we sort of think of as what can be automated versus what needs someone to look at it?
Hemant: You've put your finger on an very important awareness in the AI community that for sophisticated, particularly business-to-business AI use cases, the best practice is increasingly machine first, human in the loop. You mentioned some of the metrics on our fundraising so far. A lot of that investment is to fund the AI work to define the machine learning to perform these extractions which, of course, human experts have to curate, train the machines. It's not magic. You have to curate the transformations. There's a constant investment in automating more and more of the process, but you need to continue to have humans in the loop to identify and flag and resolve the border cases. A lot of the tech process is not only automating the extractions, it's systematizing when you need to alert a human to either intervene, check, verify the transformations that are taking place because this is critical information and machines are not perfect. They're only as good as the data sets. You have to train them and the humans that are able to curate them.
Now, you start to see enough data, you can automate more and more. For example, one of the key artifacts that our machines are very good at automating is the processing of property conditions assessment reports. We've seen lots of PCAs, as they're referred to. Every time a property owner acquires a commercial asset, they commission a property conditions assessment report as part of their due diligence process. Each one is different but they're similar patterns, and we've seen thousands and thousands and thousands of them now. Actually, tens of thousands, and so the machines are getting pretty good. Now, there are some more novel data sets where we go straight to human to review because we're still curating. So there's a range of practice but the mindset and the methodologies are machine first, train your machines, and then keep 'human' in the loop to resolve the edge cases.
Matthew: Yeah. I guess the big difference of what you are doing is because you're going right upstream and working with the originator of the data, the data is there as opposed to an insurance company where it's just not there so they've got to then go to figure out how to fill the gaps. Who's driving this now? Are you finding you're getting a push from the risk managers who are recognizing the benefits of this and are actually pushing it out or is it more of a pull from the insurers and the brokers who are seeing the benefits of doing this?
Hemant: Our initial insight was informed by the experience of the underwriters because that's... As you and I, we share that background where we did a lot of work with insurance companies helping deploy models, helping them ask for data to power those models and their constant frustration with the challenges of getting quality data consistently formatted that they can rely on to power all those downstream modeling, whether it's for CAT or attritional or all other peril rating engines. That was the motivation. Our insight was, "Let's go upstream to the owner and embed a system of truth in their own data as the owner, developer, manager of these assets and initiate the flywheel from essentially the outside in, from the customer, the end buyer, to the broker, to the underwriter." The driver of the flywheel for us has been by starting this process with the large owners. Some of the largest buyers of commercial property in the world are already on Archipelago, so we have several trillion of a unique mature value of commercial property on the platform.
But what's interesting that's been happening in the last year is you start to see the feedback loops turning. I was just talking to a senior insurance executive who was commenting that more and more of their submissions are coming in on Archipelago and now they're talking to some of their brokers to encourage them to bring more submissions like this because it makes it easier for the underwriters to do their job.
They're now encouraging the brokers. Every one of our customers, top-tier owner of property and buyer of commercial property, has a top-tier broker. Those brokers are now saying, "Hey, we have other customers that are not your customer, Archipelago, that might benefit from this process."Now we have... Several brokers are actively onboarding their customers onto Archipelago or introducing us directly to their customers to form a direct relationship.
At first it was the hard work of one by one getting the large owners to adopt, onboarding their data, supporting their submission, and now we're starting to get underwriters and brokers providing feedback loops that are starting to pull more data sets in, which is really encouraging because you can see a system can tip pretty quickly. You don't need to have 51% adoption before a system tips from one state to another. We're starting to see the first real concrete evidence of these network effects pulling in additional data sets and customers onto the platform.
Matthew: Yeah. Well, we've posted that before somewhere else. The property owner, so they have a choice, don't they? I mean particularly now. We can talk a bit about captives in a minute, but they themselves are getting more sophisticated, getting more power about how and where they buy their insurance versus retaining it internally. Presumably, that's been part of the driver behind this so they get more insights, and then they can go and have discussions with their insurers about what their options are or their brokers about what their options are for how they buy the coverage.
Hemant: Yeah. With all things, you need to tap into a larger flow or forces that are at work in order to change business practices, particularly in deep complex B2B verticals like property and insurance. In many ways, we are tapping into a growing desire from the large property owners and property insurance purchasers to take control of their own view of risk. We hear that phrase a lot or variations on that phrase where they want to shift from, "My role is to buy insurance," to, "How do I think about my role being? Maybe I'll sell some risk." Rather than, "My risk is... Somebody tells me what my risk is in the underwriting process," to, "I know what my risk is and I will decide whether to transfer or not if I think the market is advantageous and offering good terms to do so."
Some of this manifests in captives and some of this manifests in retentions. Some of this necessity is the mother of invention where with rates increasing, having to retain more risk, powering more motivation to understand what it is. But we do see this shift, particularly amongst the large owners, where they have increasing motivation to own their own view of risk, manage their own risk, yes transfer risk but have the data and the insight they need to make these decisions proactively. This is also being accelerated by other forces such as the increasing drumbeat of ESG+R initiatives, where there's other stakeholders besides insurance that are increasingly interested in how these large property owners understand, manage and mitigate their physical climate risks. It all is conflating into a similar set of motivations which is, "We as the corporation who actually owns these assets or operates critical business activities out of these assets, we need to understand our own view at risk. We need to manage our risk, and yes we transfer risk but the operative function isn't the purchase of insurance, it's the management of the risk." This starts with the very large corporations. Some of them have balance sheets that rival the size of the whole US insurance industry, but we're seeing it more and more, this drumbeat of buyers starting to shift their objectives and their mindset to be more proactive. A consumer of insurance is part of a larger context of managing their own view of risk.
Matthew: Yeah. I had a really interesting discussion with Richard Cutcher who runs the Global Captive Podcast, which is episode 198, and I certainly learned a lot from that about captives. It definitely echoes what he was saying, and also the size of some of these companies as well. They're very well capitalized and can absorb a lot of that lost potential on their own balance sheets, but also, actually, the other thing he mentioned was there's starting to be a sort of recognition from these companies about the sophistication of the analytics they can use to measure the risk. I mean, it's almost by definition, the people you're working with are already understanding what you are offering in terms of helping them on the analytics and data side. But just in terms of perspective, what you've seen over the years because you've known the kind of corporate world for more than just the last four years since you were in Archipelago, are you sort of seeing a dramatic shift now in how these companies are thinking about the use of data and analytics for their own risk mitigation and risk transfer?
Hemant: Well, there's definitely a kind of secular shift happening. In many ways, if you look at the first wave of analytic adoption that you and I helped catalyze on the modeling side, in many ways it started with the reinsurers and then it moved upstream to the insurers. Now we're seeing it move upstream to the large buyers who historically have been on the outside of this process increasingly saying, "I need to be inside this process in no small part because I have a large captive. I'm my own insurance company. I have to have the same capability to understand exposure and risk and make rational decisions as my insurers do."
So yes, we are seeing a remarkable shift and I do think it's already approached a tipping point. Part of it is catalyzed by some of the hard market of the last several years, but part of it is being catalyzed by some of the governance kind of forces on these companies, and some of it is being catalyzed by it's now possible for them to do so. The tools, the data sets, Archipelago makes its contribution, others are as well, to making it more practical for these large corporations to not just rely on their insurance partners to inform them what their risk is as part of the placement and underwriting process, but actually have a proactive view and make decisions themselves about how they want to retain, structure, mitigate and, yes, transfer risk whether to captive, traditional, insured and layered programs, alternative forms of risk transfer like catastrophe bonds. More corporations are... There were several corporate CAT bonds issued last year. All of them were associated with Archipelago customers. Perhaps a coincidence, perhaps not. But yes, they're exploring multiple proactive strategies and that has changed in the last few years. Seeing that change is part of what motivated me to start Archipelago, that the time is now ripe to make this shift.
Matthew: What about some real-life case studies or clients you can talk about just to bring this to life?
Hemant: One company that I can discuss publicly that is going through this journey is Prologis. I think we chatted briefly about Prologis last year but since we've last spoken, Prologis issued an indemnity cap bond as a corporate buyer. One of the key enablers of them to do so was they have their data sorted. We know one of the key issues with corporates and cap bonds is that, yes, parametric deals are available and have a lot of merit, but then they have to retain the basis risk. When you're an individual corporation versus a reinsurer issuing a CAT bond, basis risk becomes more and more of a concern. Indemnity deals relatively are quite attractive and desirable because it removes basis risk, but to do an indemnity CAT bond on a corporation you need to have great data. You can't be ambiguous on the locations of your properties, you can't be ambiguous whether it's masonry, non-combustible, tilt-up concrete, or dual steel frame brace system. Those need to be precisely coded and the investors need to be confident that there is no uncertainty or minimal uncertainty in the underlying data sets that power these indemnity bonds.
That's an example that Prologis was able to execute a sizeable indemnity deal in no small part because they had verified, enriched their data with evidence links, and we helped them do so and that gave them an extra tool in their risk transfer tool kit that they previously didn't have.
[Editor’s note: read customer case study with Prologis.]
Matthew: Just for those who aren't aware of what an indemnity deal is, that's basically a transaction which is mostly conventional insurance when the payout is based on the loss. To your point, you can understand that modeling when you've got good data. Don't want to be too much a downer, though, for all our friends now that are developing parametric solutions. There are still are plenty of opportunities in parametric, and in fact, parametric solutions are also helped by better data. But it is an interesting... I mean, look at the percentages of deals done on the catastrophe bonds or the insurance bank securities. I think about 15%, we reckon, were actually parametric and still above indemnity.
Ali Smedley: Hello, Ali Smedley from the research team here. ESG and climate change are topics that are becoming more important than ever. We wanted to explore what we could do at InsTech to limit our own environmental impact. We have calculated the carbon emissions caused by the lighting, heating, air conditioning, and general running of our event venues. By working with Ecology, we've supported a solar electricity project in Egypt, protection of the Amazon rainforest in Peru, and a hundred trees being planted all to offset our event emissions. We've done this for all of our events we've held this year up until October. We look forward to continuing to support this initiative.
Matthew: I want to come back to this point you made last time. One of the questions I had for you was, "How rich is the data from other data sources coming out? Are people using sensors and things?" Your answer was, "Well, in some cases, many cases at that point, many risk managers didn't even know what properties they're insuring let alone getting down to the level of detail about what those buildings are doing or sensors." Are you seeing that trend improving? It sounds like that's what you're saying but just to sort of validate that so that now your clients or the people that are going to become your clients are actually getting a better understanding of what's in the portfolio and then, to your point, is now the work is actually to get to more resolution around the data itself.
Hemant: Yeah. I think when we chatted last... It is very appealing and exciting to talk about novel new sources of data like sensors, and we do so with our customers, and this tsunami of digital living data that has no analog in the spreadsheet is a very compelling and important topic, how to enable that, how to connect that data, how to process and interpret that data. I was also cautioning that a lot of it is more blocking and tackling, that there's a lot of... I'll call it lower hanging fruit, need for improvement around, before we dive into the topic of sensors, around understanding is the portfolio up to date? Because it may seem... It's easy to throw stones, but it is a complicated problem. Some of these large property owners, they're buying and selling property all the time. An individual unit of building may not be changing every day but every day their portfolio is changing. It's not just because adds and deletes, acquisitions and divestitures, roofs are being replaced, loss control engineering reports are being performed and recommendations are being implemented.
At a portfolio level, these things are changing all the time. When we started we weren't sure does this data even exist? We're seeing these patterns where, yes, property exists along a lifecycle. It's built, it's maintained, it's prepared, it's mitigated, it's insured, it's inspected. At each point along the similar lifecycle, different sources of data get created, have nothing to do with the insurance proposition. They already get created because there's other business needs for that data and you can tap into that flow and design machines to extract that data to create a high-quality data set and do that in scale. When we first got started, it would take us months to onboard a large company's portfolio. Now we can do it in weeks and we'll get it down to days. This process is working, which is giving me confidence that we can now begin to talk about what happens next. Improving the process around the data is important, but that sets the stage for what comes next.
Matthew: Okay. Well, let's not go there yet otherwise you've got to end the podcast and I've got some more questions because that was your final question, "What happens next?" But to your point there, I mean, of course, insurance isn't existing in isolation. There's prop-tech and there's... I'm sure there's loads of other techs. There's adjacencies where data's being created anyway. It sounds like the answer is certainly getting better in terms of that risk manager not knowing where his or her property is, but we're not there yet. I think the whole topic of digital twins is fascinating, but the point is if the data you really need to make a decision is not there then all the interesting variable data is actually not very useful because you still need the fundamental data to make a decision. That's a good direction of travel, but my warning to anybody in that area is don't get too carried away with the vision of digital twins if you're missing some of the stuff that's going to determine the decisions the underwriter's going to make.
You mentioned climate in there as well and you said ESG+R, is that right? Is that... I know... Well, I think everyone now knows ESG – environmental, societal, governance. What's the R you added in the end?
Hemant: I'm starting to hear more of the R, read more of the R and discuss more of the R, as we're discussing here. Increasingly, ESG, which is widely discussed, is being augmented by ESG and R as in resiliency.
It's about resiliency. Physical climate risk is an important dimension of... Now increasingly important dimension of ESG and ESG+R imperatives.
Certainly, there's a fair amount of scrutiny on institutional owners of commercial real estate are being asked as part of the ESG increasingly, "How do you assess, benchmark your resiliency of your property, and report that to your investors, your limited partners, your stakeholders?" It's now increasingly of interest to the operating companies as well and not just to people in the commercial property business, but large corporations who happen to have property as a key part of their operating system, if you will, for the business functionality of their business.
Resiliency is coming up more and more as a key imperative not just in the context of insurance. Yes, you want a highly resilient property as in it's not vulnerable. It's also resiliency as in the resiliency of the property as it speaks to the resiliency of the business. Stakeholders are increasingly looking for reporting and insight from these corporations on, "How do you think about resiliency? How do you measure resiliency? How do you improve resiliency? What are you doing to do so?" This is adjacent to insurance, but it reinforces the same imperative, which is to answer these questions. It's not only about answering these questions so you can produce a high-quality statement of values to get your insurance renewed, it's increasingly about answering these questions so you can manage the resiliency of your business to natural hazard and climate risk.
Matthew: In my view, as I say, you'll share this, is that insurance has done probably the best of anybody to be to understand risk in the long term. I mean, this is partly going back to probabilistic nature of catastrophe modeling, but that, I think, will continue to be the state with looking at the whole ESG and the climate. I don't want to start talking about climate condition models and sort of forecasting the future state. We could probably do a special podcast just on that because I'm sure you're looking very carefully at what's happening on that.
But just back on that point about the information coming out because... I mean, from an insurance perspective in simple terms it's like these three areas they're getting asked to report on, ESG. One is on the underwriting basis, and particularly in the UK the Bank of England is actually explicitly asking people to model future scenarios. The second one is from investors looking at it from the portfolio. The third one is this whole transition risk and looking at new areas of underwriting.
Then itself, you've got a pretty big market there just purely within insurance, but you now also start to sort of get questions from your clients where they – you kind of hinted at this – but where they're being asked by totally different sort of groups of people to report on the risk, "Is this going to be a loose brick." Getting into investing and banking and the capital markets for maybe using some of the historic approaches, or it's not as much historic, but the well-tried approaches for looking at extreme events and actually those starting to enter themselves into that new area of sort of finance.
Hemant: Yeah. It's coming up a lot, and often what we hear when we talk to our customers is that, when they look at their insurance process, they see a lot of detailed modeling. Now, it still feels too black box to them, but they understand that they're these models, they're the data that matters, it's important to collect this information. There's a very analytical data-driven process, warts and all, as we've been discussing, with the data, but it's a reasonably well-underserved process. Then they look to the other side to these resiliency imperatives and they scratch their heads and they say they feel very unclear on how you measure...
"What are the metrics? Why are these metrics different than the insurance metrics? Can we have a unified framework for thinking about this?" They're like, "We want a unified framework. We don't want one framework which is about reporting to a regulator, another framework which is reporting to your investors, another framework for reporting to your insurers."
These things all exist on a continuum and it's about the underlying risk and resiliency of the property, and we should be able to make data-driven decisions along that continuum in a completely consistent fashion. The choice we make on whether to form and structure a captive or buy an extra layer of insurance is the same process we should be going through to decide, "Do we actually mitigate the asset to improve its resiliency for reporting to a key stakeholder?" That's an operational consideration. Or, "What do we want to factor into our due diligence when we bought the building in the first place?" These should all be a continuum of decisions driven by common data and common frameworks. Right now, there's a real balkanization and I'm hearing more and more of our customers saying, "Hey, can you help us think this through? Because you clearly are helping us think through our data." You are thinking through the data through the lens of risk and risk management so stay tuned on that one, Matthew.
Matthew: Hemant, I want to come back to another point that you made. You've touched on it a little bit but the challenge of change in an industry, particularly one where there's no external forces, or maybe there are external forces, to drive this. Actually, another former colleague of ours from RMS, Steve Petri, and I were talking about this a few weeks ago about... Jim Collins has this great quote that you need to create technology that, I think you can translate it to most businesses, that's actually 10 times better than what people are using to get them to shift. I mean, there are some external factors in here, but you've got investors who've put a lot of money at the company, they generally look for quite speedy returns. How do you accelerate that change? If you just let it progress its natural momentum, I suspect it's not going to be fast enough so just interested in what techniques you've found or other external factors that are driving change.
Hemant: Well, first I'll embrace the underlying challenge that you've outlined, which is changing prevailing practice in traditional complex industries is very hard. Sometimes people come to these things naively and think, "Oh, the existing practice doesn't make any sense. There's a better way. I've got an algorithm, a hoodie, a pair of cool sneakers. Presto, it's going to change."
If it was easy to change, it would've changed a long time ago. Part of it is deeply respecting the status quo ex-ante and understanding why are things the way they are. It's not because people are lazy or dumb or not innovative. Often, these processes, these practices have built up over years and sometimes generations for very good and logical reasons. If you don't understand that, you can't change the system. You have to embrace it, understand it, meet it where it is, and then move it systematically.
I think that has been a big part of the Archipelago mindset which has been appealing to customers because when we show up, we understand and we convey a deep appreciation and empathy for the current pain points. We understand why they've things have developed the way they are. We're not naive in our understanding of how things should be better so we meet them where they are and we have a systematic approach to changing this in a logical way. One of the key drivers of how to change systems is to stimulate these network effects that we were describing, and that has been a key part of our strategy which is, yes, let's start with changing behavior in what sometimes some investors call single player mode to go to these large top tier owners, like a company like Prologis, I mentioned, or the largest tech companies is on our platform as well or one of the largest owners of hospitals.
Except these top-tier, what we call eagles, these are not only very powerful brands, they're large buyers of commercial property insurance, are well-respected companies, they have important trading relationships in the market and you get them on board. When you get them on board in single-player mode, they invite in their broker partners who get to see the benefits. Those broker partners invite in the underwriters who get to see the benefits. You've got to cultivate those feedback loops to create the dopamine loops that start to change people's perception of what's possible.
Once you start to change the perception of what's possible, people start to think, "Well, this could actually change." They start to believe. If they see it's possible and they see it changing, they can envision, "Well, this could really change." If they think there will be really change, they start to lean in and contribute to that change because they can believe it can change.
You have to first demonstrate you can start the flywheel. Then you need to engage people, "Oh, I believe this can actually change and then I'm going to contribute to that change." It's a journey. It's a hero's journey.
The hero is not Archipelago, the hero is the underwriter, the hero is the risk manager, the hero is the broker.
Engage people emotionally, that there's a journey that they can take to contribute and be part of the change and that's a lot of the art of change management and changing these systems. So yes, there's an analytical aspect which you have to create massive ROI. Sometimes people are like 10x ROI to get something to action from one state to another, but it's also engaging the people to see themselves as part of this journey and get them excited and emotionally engaged, that, "This is a better way and I want to be part of it so I'm going to advocate."
One risk manager advocating to another in a forum, which has happened a lot for us, a risk manager advocating to their broker who then advocates to one of their other customers, the broker advocating to the underwriter who then advocates to their other broker who advocates back to their customer that this is a better way, it's practical, it works, it's possible. A lot of it is you have to create people. You have to get people engaged in, "It's possible. You can do it." Then they can actually contribute to it as opposed to watching it. They can actually help accelerate it, and that's the change journey that we're going through across this three-part ecosystem with these mutually reinforcing feedback loops.
Matthew: Eagles in single-player mode. If I don't remember anything else in this podcast, and of course, I will, it's going to be eagles in single-player. But I also noticed you managed to slip another Jim Collins quote in there. Well done. You get an extra point for that with your reference to the flywheel from good to great. For those that have made it this far, can you now reveal in however much detail as you want to... When we're talking again in a years' time, what are we going to be talking about that you'll be doing in the next 12 months?
Hemant: Much of it is what we've just alluded to, which is this tipping point of expectation where because of the network effects over the next year, we're going to go from a state where an underwriting team getting an Archipelago-enabled submission is going to shift from, "That's great, I'm excited." To, when they don't get one they're going to be, "What is going on here? Will you please not send me another spreadsheet?" When that sentiment tips from, "I'm surprised and delighted," which is great, I love that. That helps validate that we're doing something right. To the expectations, "I want to now see most, if not all, the submissions because it's possible I've seen enough. Now my expectation has tipped from I'm surprised and delighted to I expect it and there might be consequences if I don't get it this way." That's kind of one important shift.
Another is that we're going to be able to shift the conversation from talking about the process of sourcing, connecting, validating, and then sharing high-quality data sets, both exposure and loss, to what's the insight that's coming from the aggregations of that data.
That's a whole other layer of what we're setting out to do very openly because it's not just that the data is inefficient, it's not as accurate as it should be and that leads to suboptimal outcomes for everybody. It's that the whole system is very opaque.
When you start to get trillions of dollars of data and millions of properties, you start to see patterns in the data. That sheds more light, that creates opportunities to make the system more effective.
Yes, definitely humans' judgment, experience are always going to matter, but there are opportunities for more machine-driven processes across this whole value chain that will lead to better outcomes for everybody. The aggregations, the data will start to show some of the patterns that suggest the way forward on that front as well and that will happen within the next year.
Matthew: Well, thank you for your support for InsTech as well. I look forward to seeing what you're doing. Yeah, now we're allowed to travel again we either will come out to California or we might see you in London. I'm sure you'll be over here before too long.
Hemant: Be wonderful to see you in person, Matthew.
Matthew: Thank you very much.
Hemant: Okay. Thank you.
Matthew: That was a lot to catch up on so I'm not going to hold you up for much longer. Everything you want to know about us is at www.instech.co. Please don't forget to tell us what you think. Message me on LinkedIn or comment on this episode or better still, tell your friends. That's it. We're done.
Share this
You May Also Like
These Related Stories
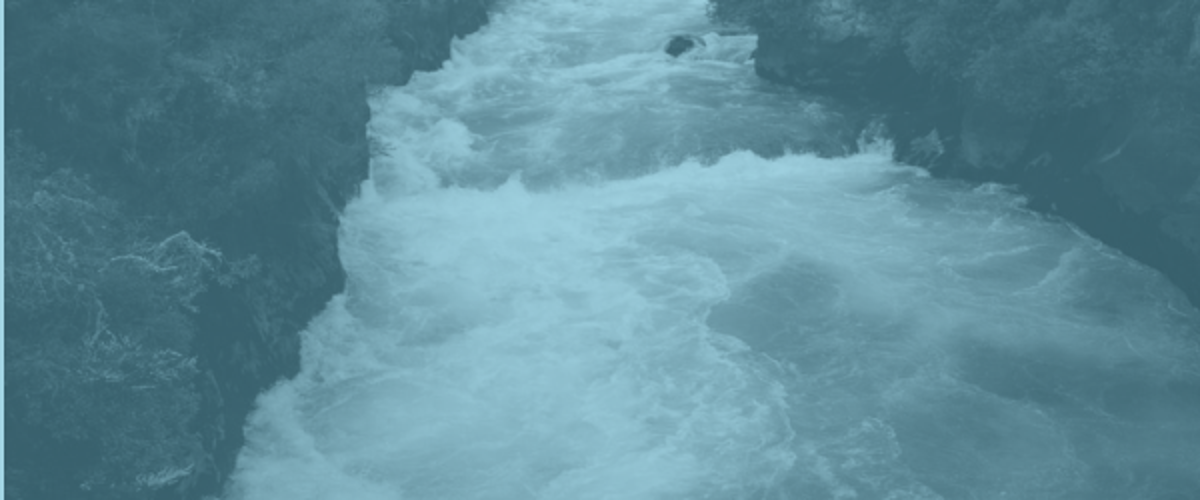
Moving Upstream: Digitizing & Standardizing Customer Data at Source
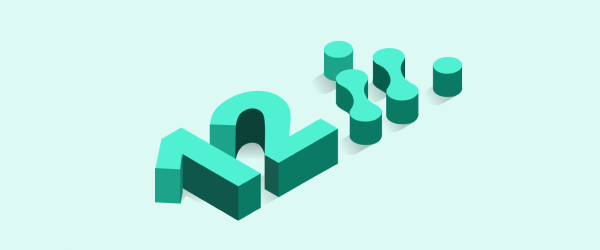
12 Qs with Mark Cravens, Principal at Cravens Consulting
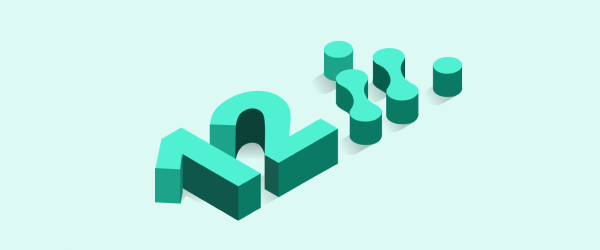